The large number of devices connected to the Internet has led to exponential growth in data. IoT (Internet of Things) technology has enabled communication between humans and devices as a key element in making rapid and revolutionary decisions. Data collected from multiple IoT devices has value in itself that is difficult to extract due to its complexity and nonstandard nature.
To recover and amplify business value, IoT data must be combined with existing non-IoT data. The optimal solution to manage both, efficiently, is to create a modern cloud data lake and use established best practices to prevent it from becoming a “data swamp.”
Data Lake in cloud
Data lake storage in the cloud is transforming the way we think about data lakes. Cloud storage solutions, such as Amazon S3, have introduced new features, such as infinite scalability, cost flexibility, ease of maintenance, and high availability. These features have changed the perception toward data lakes, which are no longer seen as just a backup tool, but instead are the place where data arrive and are organized.
Data lake storage has also been a game changer as far as data processing is concerned, because you can now benefit from the separation of processing and data. Less than a decade ago, there was talk of bringing processing to data with the goal of running everything in the same cluster and getting faster performance as a result. In the cloud, things work differently, not only because the network infrastructure has improved, but also because of the separation of processing and storage.
This means that it is now possible to have storage as a service and separately leverage pipeline services and compute engines, such as Spark and Hive, that can run directly on the data. This is a key benefit for IoT data lake infrastructure because you can scale data and processing separately, providing granular control over speed and cost.
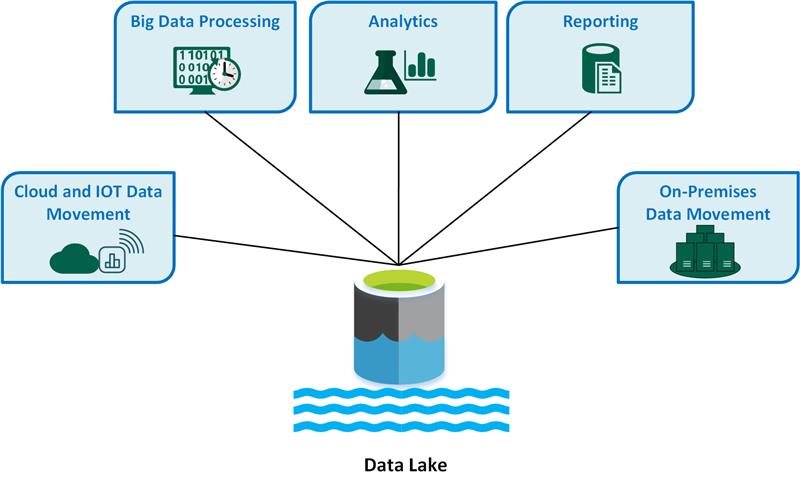
Data Lake and IoT
In traditional data warehouse approaches, data obtained from IoT devices must be transformed, standardized, and blended before it is ready for analysis. This process is slow and expensive and can lead to missed business opportunities. To do it right, we need to learn how to simplify the process by building a data lake that is flexible, fast, secure and cost-effective.
IoT infrastructures generate data of different shapes and sizes, and although extraction, transformation, and loading processes are still required to get data into the “lake,” it is possible to avoid having to implement similar processes when delivering data to end users. This ensures direct access without costly de-serialization of the retrieval of saved data.
To learn more about data lake topics, read our article HERE